Data annotation is essential for AI/ML success, providing accurately labeled data that improves model performance. It supports tasks such as image recognition, NLP and video analysis. By ensuring high-quality annotation, businesses can enhance AI accuracy and drive better decision-making outcomes.
More often than not, the reason for underperforming machine learning (ML) and artificial intelligence (AI) models is poor quality data. If you’ve experienced inconsistent results or models that fail to meet expectations, the root of the problem usually lies in the data itself.
Contents
- What is Data Annotation?
- The Role of Data Annotation in Machine Learning and AI
- Types of Data Annotation Techniques for AI/ML
- Common Data Annotation Challenges in AI/ML Projects
- Best Practices for Effective Data Annotation
- Key Benefits of Data Annotation for AI/ML Projects
- The Future of Data Annotation: Trends and Innovations
- Conclusion
Data annotation for ML and AI plays a key role in transforming raw data into structured information that models can learn from. Without accurate annotation in machine learning, even the most sophisticated algorithms struggle to deliver reliable outcomes. From image recognition to natural language processing (NLP), well-annotated data is the key to unlocking the full potential of AI systems.
Partnering with an experienced data annotation service provider can ensure that your data is meticulously labeled, enhancing the overall performance of your models. In this blog, we’ll explore why data annotation is essential for improving the accuracy and performance of your AI models and how it directly impacts the success of your projects.
What is Data Annotation?
Data annotation is the process of labeling raw data, such as text, images, audio and video so that machine learning (ML) and artificial intelligence (AI) models can interpret and learn from it. In the context of AI/ML development, this labeled data serves as the foundation for models to recognize patterns, make decisions, and generate accurate predictions.
AI data annotation involves identifying and tagging specific elements in datasets. This includes tasks like labeling objects in images, highlighting key segments in text, identifying key sounds in audio files or tracking actions across multiple frames in videos. This structured transformation allows models to learn effectively from the data and continuously improve over time, ensuring the accuracy of annotation.
Types of data annotation in AI include image annotation (e.g., bounding boxes for object detection, semantic segmentation for pixel-level accuracy), text annotation (e.g., sentiment analysis, named entity recognition), audio annotation (e.g., speech-to-text transcription), and video annotation (e.g., activity recognition across multiple frames).
Each annotation technique plays a critical role in developing AI systems that depend on a precise and efficient data annotation workflow. Ensuring annotation quality control is essential for producing robust models that function well in real-world applications.
It is important to start from the basic of data annotation for AI to provide the foundation for building advanced AI models that require high-quality labeled visual data.
The Role of Data Annotation in Machine Learning and AI
Data annotation is critical for the success of machine learning (ML) and artificial intelligence (AI) models. It is especially important in supervised learning, where labeled datasets provide the essential training ground for models to learn from. By offering structured, labeled data, data annotation for ML and AI enables algorithms to recognize patterns, predict outcomes, and deliver actionable insights. This labeled data is essential for diverse AI applications, such as computer vision, natural language processing (NLP), and speech recognition.
The Process – The data annotation process involves several important steps to ensure the accuracy and reliability of labeled data for machine learning (ML) and artificial intelligence (AI) models.
Here are the steps:
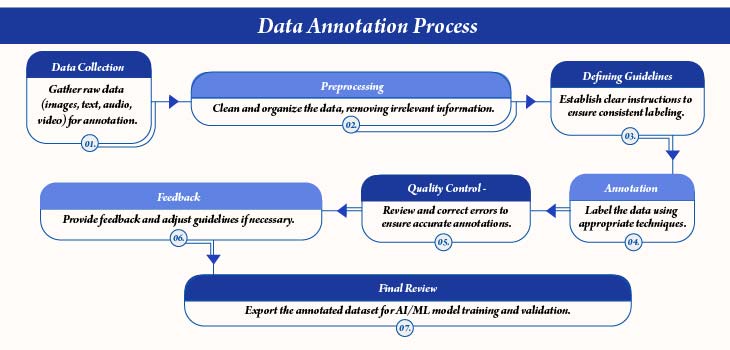
Experts – The role of data labelling and annotation experts is crucial in this process, as their domain knowledge and attention to detail help ensure accurate and contextually relevant annotations, which directly impact the quality of the AI models. These labeled datasets are used to train machine learning models, which then use these annotations to identify patterns and generalize from training data to unseen datasets.
Quality – The quality of data annotation directly correlates with model performance. High accuracy of annotation ensures that the models produce reliable results. This is especially important in complex AI tasks, such as NLP models or recommendation engines, where personalized experiences depend on detailed data annotations. Well-annotated datasets also enable AI systems to generalize better, improving their adaptability and reliability across various real-world scenarios.
To achieve these outcomes, maintaining an efficient data annotation workflow and rigorous annotation quality control are essential. High-quality, well-labeled datasets enable AI models to function at peak performance, ensuring accuracy in predictions and the ability to scale across diverse tasks and industries.
Types of Data Annotation Techniques for AI/ML
To build effective AI/ML models, different types of data annotation techniques are employed, each tailored to specific tasks, such as visual recognition, autonomous systems, or natural language understanding. Let’s explore the key techniques that drive AI advancements.
Image Annotation
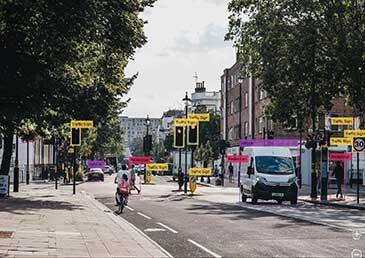
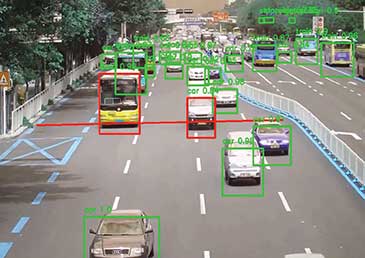
Used in computer vision for object detection, facial recognition, and autonomous systems. Image annotation techniques like bounding boxes, polygons, and semantic segmentation label objects within images, enabling models to interpret visual data accurately.
Example – Labeling objects in an image, such as drawing bounding boxes around cars and pedestrians in a street scene for autonomous driving applications.
By partnering with HabileData for data annotation, the client enhanced their machine learning models for accurate food wastage analysis. The detailed annotations provided helped improve business performance, allowing the client to assess food waste more effectively and build credibility in the market.
Video Annotation
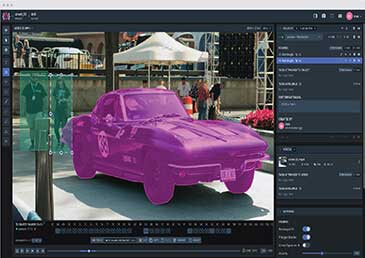
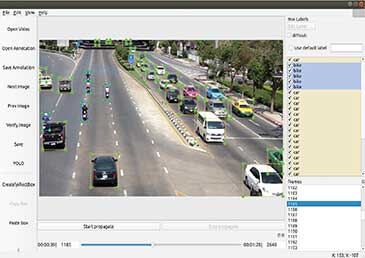
Essential for dynamic data, this technique labels objects or actions across multiple frames. It’s widely used in autonomous vehicles, surveillance systems, and robotics for tracking movements and predicting activities. By annotating objects or individuals in motion, video annotation enables models to track and predict movements, recognize activities, and make real-time decisions.
Example: Tracking the movement of a person across multiple video frames, labeling actions like “walking” or “running” for surveillance or activity recognition models.
By annotating both pre-recorded and live video streams of vehicles, HabileData provided critical training data for machine learning models, enabling a California-based data analytics company to enhance traffic management. This video annotation solution improved traffic flow analysis, safety, and operational efficiency through real-time categorization and annotation of vehicles.
Text Annotation
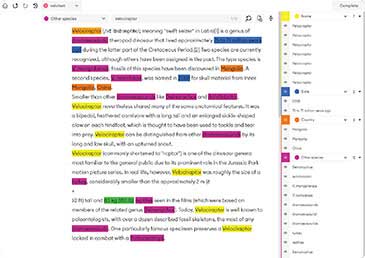
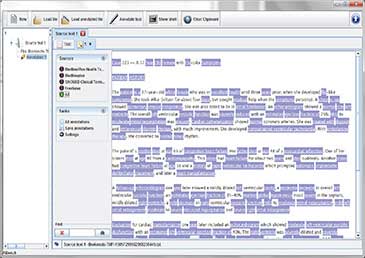
Critical for natural language processing (NLP), it involves labeling entities, keywords or sentiments within text. Applications of NLP data annotation include speech-to-text conversion, document classification, and language translation, where correctly labeled text data is important for accurate model output.
Example: Tagging named entities (like “London” as a location or “Google” as an organization) in a news article for natural language processing (NLP) tasks like entity recognition.
A perfect data annotation workflow helped the client significantly reduced data processing time and improved the accuracy of their AI model. Over 10,000 construction-related articles were annotated and verified, enhancing customer acquisition and cutting project costs by 50% while enabling more precise data analysis for their real estate platform.
Text annotation is a crucial step in preparing data for Natural Language Processing (NLP) systems. It is important to understand how annotated data powers NLP models.
Each of these data annotation techniques plays a significant role in the development of AI models across industries, ensuring that AI/ML systems can interpret, analyze and respond to data in a meaningful way.
Common Data Annotation Challenges in AI/ML Projects
In AI/ML projects, achieving high-quality annotations is important, but common challenges in the data annotation workflow can significantly impact the success of your models.
-
Data Volume and Accuracy –
Managing large datasets while maintaining annotation accuracy is a major challenge. As dataset size increases, ensuring consistency in the data annotation workflow becomes harder, making annotation quality control crucial to preventing poor model predictions.
-
Human vs. Automated Annotation –
Manual annotation is accurate but costly and time-consuming. Automated systems handle large datasets quickly but struggle with complex tasks like medical imaging or NLP, requiring a balance between manual and automated efforts.
Integrating a Human-in-the-Loop approach can significantly boost the accuracy of annotation , ensuring both speed and accuracy.
-
Ongoing Data Refresh –
AI/ML models need constant updates with newly annotated data to maintain accuracy and relevance, requiring a continuous annotation process for robust performance.
-
Data Quality and Consistency Issues –
Inconsistent or low-quality annotations can degrade model performance. Ensuring quality across different stages and annotators is vital, especially when mixing manual and automated methods.
-
High Cost and Time Investment –
Manual annotation demands significant time and resources, particularly for large-scale projects, often necessitating a blend of human and automated workflows.
-
Domain Expertise –
Specialized fields like medical imaging require domain-specific knowledge for accurate annotation. A lack of expertise can result in poor-quality data, impacting model effectiveness.
The role of an AI training associate in data annotation is crucial for addressing these challenges, particularly in managing and maintaining data accuracy throughout the annotation process. Outsourcing to data annotation companies is a good option.
Enhance your ai with consistent annotation.
Contact NOW! »Best Practices for Effective Data Annotation
Here are some key best practices for ensuring high-quality data annotation in your AI/ML projects.
- Define Clear Annotation Guidelines – Provide precise instructions to ensure consistent labeling.
- Use Domain Experts – Leverage specialists for complex, field-specific annotations.
- Leverage a Hybrid Approach – Combine manual and automated methods for efficiency.
- Implement Quality Control Measures – Regularly review annotations to ensure high accuracy.
- Provide Annotator Training – Train annotators to enhance data quality and precision.
- Continuous Data Refresh and Feedback – Regularly update datasets for model accuracy.
- Monitor and Adjust the Workflow – Continuously improve workflow for better efficiency.
Key Benefits of Data Annotation for AI/ML Projects
Here are the key benefits of effective data annotation for AI/ML projects.
- Accuracy and Precision – Enables AI models to make accurate predictions, minimize errors, and improve overall performance.
- Improved Training Speed – Accelerates model training by reducing the need for manual corrections and adjustments, leading to faster results.
- Scalability – Allows models to scale efficiently, enabling them to handle larger datasets and adapt to new contexts seamlessly.
- User Experience Optimization – Ensure AI models deliver relevant, personalized, and smooth user experiences by improving context understanding.
The Future of Data Annotation: Trends and Innovations
Data annotation is rapidly evolving with advancements in AI-powered tools, enhancing efficiency and accuracy. AI-assisted annotation tools now pre-label data, reducing the workload of human annotators and speeding up large-scale projects. Active learning, a rising trend, focuses on labeling only the most complex or uncertain data points, improving model performance while minimizing effort.
Hybrid approaches combining human expertise with machine automation are becoming the norm. Machines handle routine tasks, while humans focus on edge cases, ensuring scalability and precision. Real-time annotation is also gaining momentum, particularly in dynamic systems like autonomous vehicles and traffic management, where real-time data labeling enables instant decision making.
AI-assisted quality control mechanisms are further improving data accuracy by automatically detecting and correcting errors and minimizing the need for manual reviews.
Looking ahead, fully autonomous annotation systems, combined with synthetic data generation, may reduce reliance on human input and accelerate AI development. These trends promise faster, more scalable, and more accurate data annotation, driving the future of AI.
Conclusion
In the development of successful AI and ML models, data annotation plays a key role. Well-labeled data form the foundation for accurate predictions, efficient training, and scalable models that can adapt to larger datasets and complex tasks. Without precise data annotation for ML and AI, even the most advanced algorithms will fall short of delivering reliable results.
For AI/ML developers and companies, investing in high-quality data annotation workflows is essential to ensuring model performance and long-term success. Whether it’s through in-house efforts or partnering with specialized data annotation for AI services, a focus on accurate and consistent annotation is key. By prioritizing well-structured, reliable data, businesses can unlock the full potential of AI technologies and stay ahead.
Power Your AI with Accurate Data Annotation
Start Your Free Trial Today! »
Snehal Joshi heads the business process management vertical at HabileData, the company offering quality data processing services to companies worldwide. He has successfully built, deployed and managed more than 40 data processing management, research and analysis and image intelligence solutions in the last 20 years. Snehal leverages innovation, smart tooling and digitalization across functions and domains to empower organizations to unlock the potential of their business data.