Data entry errors cause financial losses, disrupt operations, and lead to poor decision-making. Common mistakes like inconsistent formatting and incomplete data reduce efficiency. Implementing expert data entry solutions can prevent errors, save costs, and ensure accurate, reliable business data.
Contents
Hawaiian Airlines mistakenly charged customers in dollars instead of miles, turning 674,000 miles into a shocking $674,000 per ticket. This error not only frustrated customers, but also damaged the airline’s credibility, showcasing the severe impact of data entry mistakes on businesses.
Data entry errors can be a significant barrier to business success, leading to poor decision making and operational inefficiencies. As businesses increasingly rely on data to drive strategies, the impact of data entry errors becomes even more pronounced, affecting everything from sales forecasts to customer relationships.
Companies struggle with common data entry mistakes, including inconsistent formatting, incomplete data, and data duplication, issues that compromise the accuracy and usefulness of their data. While these data quality issues pose challenges, solutions such as data quality control measures, standardized processes and outsourcing data entry can help.
By implementing data entry best practices and automated tools, companies can streamline data capture, reduce errors and maintain high data entry accuracy. This blog explores five critical errors that companies must avoid to improve data reliability and drive better business outcomes.
The High Cost of Bad Data: Key Stats
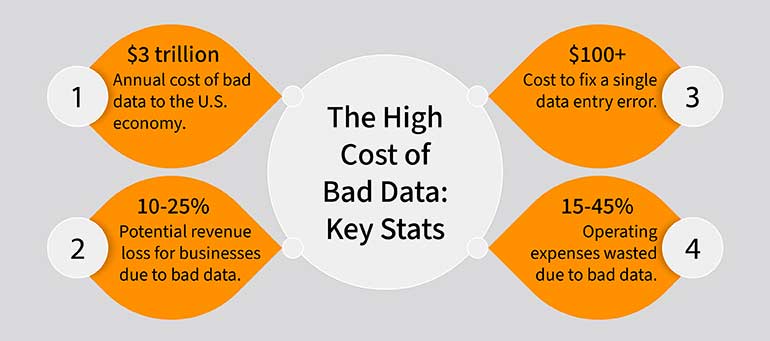
Eliminate errors and save resources with our data entry solutions.
Get a Free Proposal »Impact of Data Entry Errors on Business Success
Data entry errors can significantly affect various aspects of a business, from finances to long-term growth. Below are five key areas in which the consequences of poor data entry accuracy are most evident.
Financial Losses Due to Poor Data Quality: Data entry errors directly impact a company’s bottom line, leading to lost revenue and increased costs.
- Revenue loss: Pricing and customer data errors lead to over/under-charging and missed opportunities.
- Costly corrections: Fixing data mistakes consumes time and resources, increasing expenses.
- Financial impact: Poor data quality costs organizations $9.7 million annually (Gartner).
Utility Bills Invoice Data Entry for a Utility Portfolio Management Company in USA
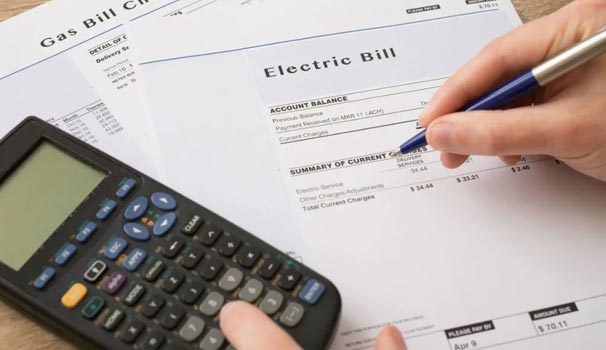
Data entry accuracy can significantly reduce financial losses due to poor data quality. For example, a Utility Portfolio Management Solutions Provider in the USA partnered with experts to handle the daily entry of 1,000+ energy invoices into a web-based application. By outsourcing this task, the company avoided billing errors and ensured smooth operations, saving both time and money while maintaining data accuracy.
Read full case study »Operational Inefficiencies: Incorrect data disrupts workflows and adds unnecessary time to manual corrections, reducing overall productivity.
- Time-consuming corrections: Fixing errors takes time away from productive tasks.
- Workflow disruptions: Errors cause delays, impacting business processes.
- Increased costs: Inefficiencies like reprocessing and shipping corrections raise expenses.
Invoice Data Entry Solution for Creating Centralized Repository
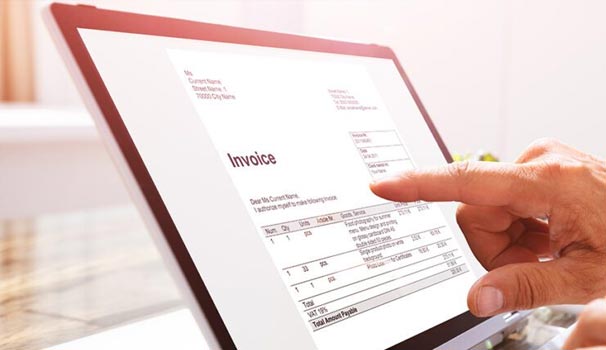
Operational inefficiencies like reprocessing and error correction often result from data entry errors. A leading logistics company addressed this by using data entry experts to convert freight invoices from TIFF files into Excel. Through double keying and rigorous quality checks, the experts ensured accurate, error-free records, creating a centralized repository and reducing manual rework and delays.
Read full case study »Poor Decision-Making: Inaccurate data compromises the quality of insights, leading to flawed decisions that negatively impact long-term strategy.
- Incorrect insights: Flawed data leads to costly strategic mistakes.
- Strategic harm: Misleading data impacts future decisions and investments.
- Missed growth: Inaccurate data causes businesses to miss key trends and opportunities.
Document Data Entry of Handwritten Scanned Images for a University in UK
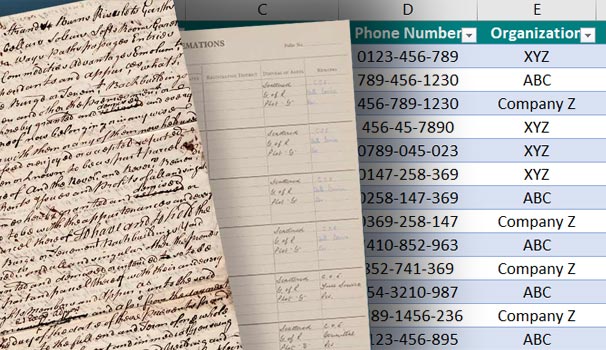
Poor data capture leads to flawed insights and decisions. A UK university needed accurate data entry for 26,000 handwritten service logs. Using double keying, data entry experts ensured 99.995% accuracy, capturing vital details like birthplaces and medical exam results. This accuracy enabled the university to make informed decisions based on reliable data.
Read full case study »Reputational Damage: Repeated data errors can erode trust with customers and partners, tarnishing your brand’s reputation and reliability.
- Lost trust: Data errors damage customer confidence and brand reputation.
- Negative impact: Errors lead to bad reviews and lost business.
- Strained partnerships: Poor data harms relationships with partners and stakeholders.
Data entry of nutrition values for buyers to make informed decisions
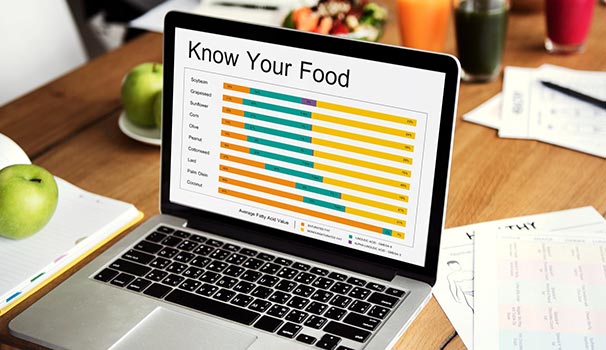
Accurate data is crucial for protecting a company’s reputation, especially with sensitive information like nutrition labels. A leading food manufacturer used data entry experts to capture nutritional values from 1,000+ product images. This ensured data entry accuracy across 36 fields, building customer trust, improving brand credibility, and driving sales. Precise data entry safeguards a brand’s reputation and supports growth.
Read full case study »Delays in Digital Transformation and Innovation: Accurate data is key to the successful adoption of emerging technologies like AI and automation, and poor data quality can stall these efforts.
- Slows AI/ML: Poor data reduces the effectiveness of AI and machine learning.
- Hinders automation: Incorrect data limits the performance of automation systems.
- Competitive disadvantage: Companies with bad data fall behind those leveraging clean data.
Protect Your Business from Costly and Avoidable Data Errors.
Consult our Expert »5 Data Entry Errors Companies Should Avoid
Now that we’ve seen the costly consequences of data entry mistakes, let’s explore the five most common data entry errors companies should avoid to ensure accuracy and protect their business reputation.
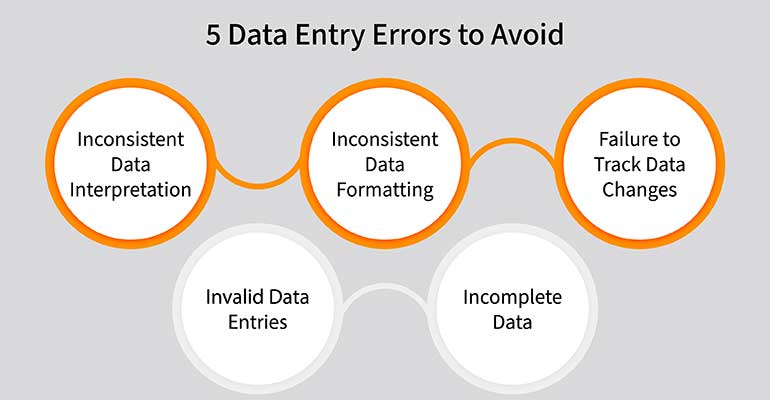
Error 1: Inconsistent Data Interpretation
Inconsistent data interpretation occurs when different departments or team members apply varying definitions to the same data, resulting in data entry errors. Without standardized definitions, this leads to conflicting reports and misaligned strategies.
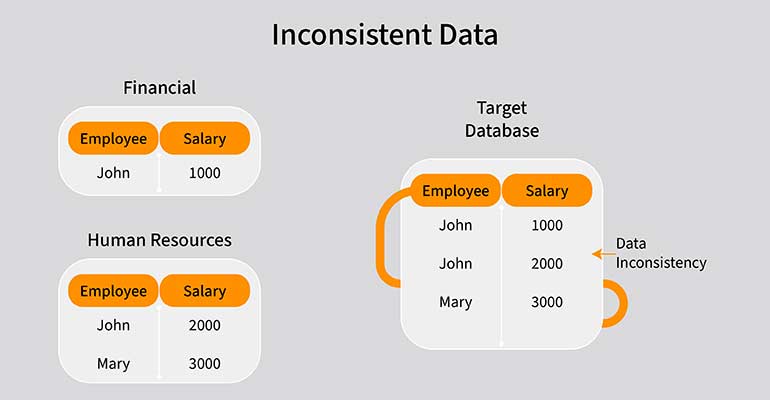
For example, in a sales team, one person may define a “preferred customer” as someone with five purchases, while another defines it by total spending. This discrepancy can result in misidentifying high-value customers and targeting the wrong audience for promotions.
Misinterpretation causes data quality issues, leading to flawed insights and poor decision making. Inconsistent definitions can also create misalignment in customer targeting and resource allocation.
Technical Impact: These inconsistencies can also affect system integration, where disparate software platforms, such as CRM systems or databases, interpret fields differently, leading to errors in data synchronization and reporting.
Solution: Implement standardized definitions for all data fields across the organization. Use a data dictionary or master data management (MDM) system to establish a single source of truth and ensure consistency across departments. Regular data audits, along with automated validation rules within systems, can further minimize misinterpretation. Additionally, provide ongoing training and clear communication around data standards to ensure proper adherence.
Error 2: Inconsistent Data Formatting
Inconsistent data formatting occurs when the same type of data is entered into different formats, creating challenges in aggregating, analyzing and utilizing the data effectively. For example, dates, phone numbers, and currency values might be input into various formats, causing confusion and errors across systems.
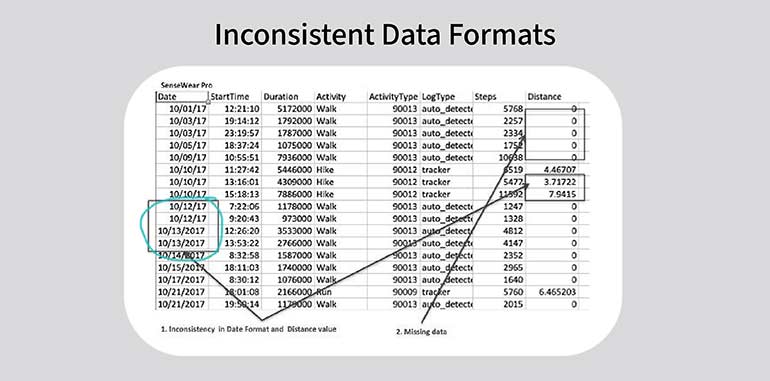
Inconsistent formatting leads to errors in data processing, making tasks like filtering, sorting and reporting inefficient or inaccurate. It also creates complications during data integration when information from multiple sources needs to be combined. This lack of uniformity can disrupt workflows, especially when systems like CRMs or ERPs depend on consistent data structures for operations.
Technical Impact: Inconsistent formatting can cause failures in data synchronization between integrated systems, resulting in corrupt datasets. For instance, during API calls between systems, fields such as dates or currencies may not match expected formats, leading to failed data exchanges or inaccurate reports.
Solution: Implement data entry standards for key fields, such as dates, currency, phone numbers, and addresses across all systems. Use automatic formatting rules and validation functions in data entry systems to enforce uniformity, like dropdown menus or predefined formats for specific fields. Furthermore, data validation APIs should be used to ensure consistency across multiple platforms and systems, especially when importing or exporting data from different sources.
Error 3: Failure to Track Data Changes
Failure to track data changes occurs when there is no system to monitor or update critical data like customer contact details, product information, or pricing. Over time, data that was once accurate becomes outdated, leading to incorrect insights and poor decision making. For example, outdated customer addresses can cause shipping errors and delays, while old pricing information can lead to undercharging or overcharging, impacting revenue and customer satisfaction.
Technical Impact: Failing to track changes compromises data integrity in systems such as CRM, ERP and inventory management platforms. Without proper version control, automated processes like reordering or pricing adjustments, can be misaligned. Additionally, the lack of historical data limits the ability to revert to previous states, thus increasing operational risks.
Solution: Implement version control and data auditing tools to track changes in key data fields. These tools ensure that modifications to critical data, such as customer profiles, inventory and pricing, are documented and traceable. Use automatic triggers and reminders for scheduled data reviews to keep information up-to-date, especially in fast-changing areas like contact details, stock levels, and pricing. Additionally, incorporate data backup and restoration systems to allow easy rollback to previous versions if errors occur, maintaining both accuracy and data integrity over time.
Error 4: Invalid Data Entries
Invalid data entries occur when incorrect values, such as an invalid email or non-existent zip code, are entered into fields. These errors are often caused by human mistakes or a lack of validation, leading to inaccurate reports, missed opportunities, and operational inefficiencies. For example, invalid email addresses result in undelivered marketing messages, while incorrect postal codes cause delivery errors, both leading to increased costs and lost sales.
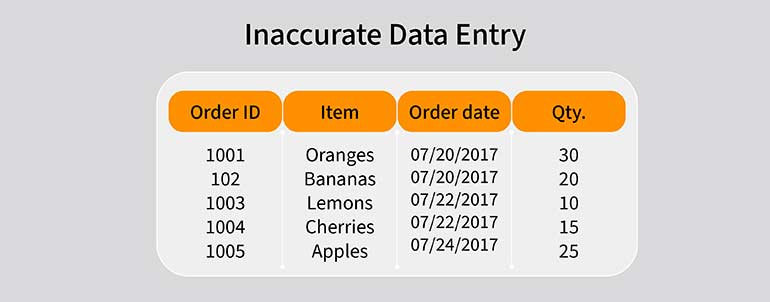
Technical Impact: Invalid data disrupts automated processes like email marketing, customer outreach, and logistics. Systems like CRMs and ERPs that rely on accurate data can produce false reports or fail to complete tasks. Over time, poor data integrity reduces the effectiveness of machine learning and analytics, which depend on clean data for accurate insights.
Technical Impact: Invalid data disrupts automated processes like email marketing, customer outreach, and logistics. Systems like CRMs and ERPs that rely on accurate data can produce false reports or fail to complete tasks. Over time, poor data integrity reduces the effectiveness of machine learning and analytics, which depend on clean data for accurate insights.
Error 5: Incomplete Data
Incomplete data occurs when key fields are left blank during data entry, creating gaps that compromise decision-making and operational processes. Missing information reduces the accuracy and utility of databases, impacting critical tasks, such as customer follow-ups, financial reporting, and order processing. Incomplete data delays business operations and can lead to missed opportunities.
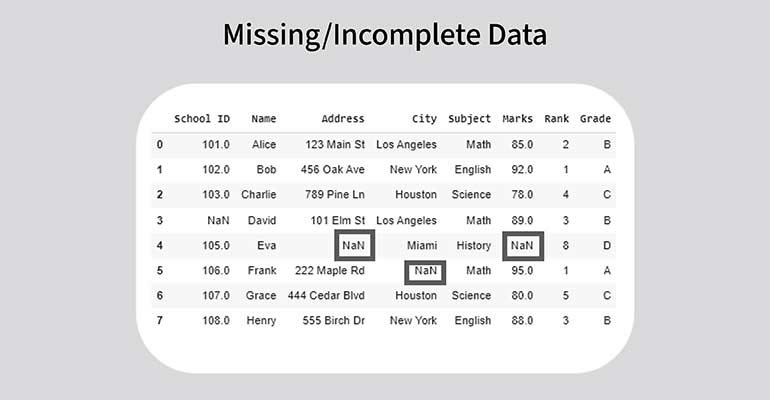
Technical Impact: Incomplete data disrupts systems such as CRMs, ERPs and inventory management platforms by hindering automation, reporting and analytics. Missing fields may prevent systems from triggering workflows, such as processing payments or sending follow-up emails. Additionally, incomplete data can affect the accuracy of machine learning models and predictive analytics, reducing their effectiveness.
Solution: Set the required fields in data entry forms to ensure that critical fields, such as contact details or payment information, cannot be left blank. Use automated prompts or validation systems that alert users to missing data before submission. Regularly audit the database to identify and correct incomplete data. Additionally, consider using data enrichment services to fill in missing information by cross-referencing external sources, ensuring your database remains comprehensive and accurate.
Impact of Data Entry Errors on Machine Learning and AI
Data entry errors can greatly undermine the effectiveness of AI systems. Poor data quality leads to inaccurate model training, resulting in unreliable predictions and diminished long-term model performance. These errors also increase the time and cost of data cleaning, delaying AI implementation and stalling innovation.
Moreover, inaccurate AI outputs erode stakeholder trust, slowing AI adoption as confidence in the technology weakens. Ensuring high-quality data is essential to maintaining the accuracy, efficiency and trustworthiness of AI systems.
How to Prevent Common Data Entry Mistakes
To maintain accurate and reliable data, businesses must adopt effective strategies to prevent common data entry mistakes. Here are key actions to ensure data integrity and efficiency:
Implement Real-Time Validation Tools
- Use real-time validation checks to flag errors at the point of data entry.
- Provide dropdown menus and predefined options to reduce incorrect entries.
- Set up immediate prompts to instantly correct invalid data.
Standardize Data Entry Guidelines
- Use standardized templates across departments to ensure data consistency.
- Train employees on proper data-entry practices to improve accuracy.
- Establish company-wide rules for consistent data entry and updates.
Leverage Automation and AI Tools
- Use automation tools to streamline processes and reduce manual effort.
- Automatically populate missing information using enrichment tools.
- Deploy AI to instantly detect and fix data inconsistencies.
Conduct Regular Data Audits
- Schedule regular audits to identify and fix outdated or incorrect data.
- Use data quality dashboards to monitor the accuracy of your database.
- Focus audits on frequently error-prone fields to minimize issues.
Establish Required Fields in Data Entry Forms
- Make key fields mandatory to prevent incomplete data submissions.
- Set up alerts to notify users when required fields are missing.
- Implement automated checks to ensure all mandatory fields are completed.
5 Data Entry Best Practices
To maintain high data quality and ensure accurate decision-making, businesses should adopt the following best practices in data entry:
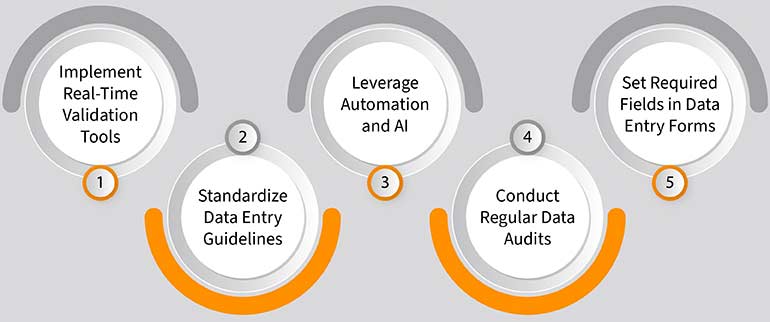
Conclusion
Data entry errors cost businesses millions annually, leading to inefficiencies, missed opportunities, and poor decision making. Issues such as inconsistent formatting, incomplete data, and data duplication are some of the common data entry mistakes that create significant data quality issues.
To mitigate these issues, companies must focus on preventing data entry errors through the use of data quality control measures and by adopting data entry best practices.
Leveraging automation and real-time validation systems can enhance data entry accuracy and minimize errors. Additionally, outsourcing to specialized data entry companies ensures that critical tasks like data capture and validation are handled efficiently. To stay competitive in a data-ruled market today, accurate data is essential for making those informed business decisions that ensure operational success.
Safeguard Your Business from Costly and Avoidable Data Errors.
Get a Free Quote »
Chirag Shivalker heads the digital content for HabileData, a global data management solutions outsourcing company, rated as one of the top BPO companies in India. Chirag's focus has been on enterprise wide data digitization, data governance, data quality, and BI capabilities.